The Impact of Agentic Human AI on Workforce Dynamics and Autonomy
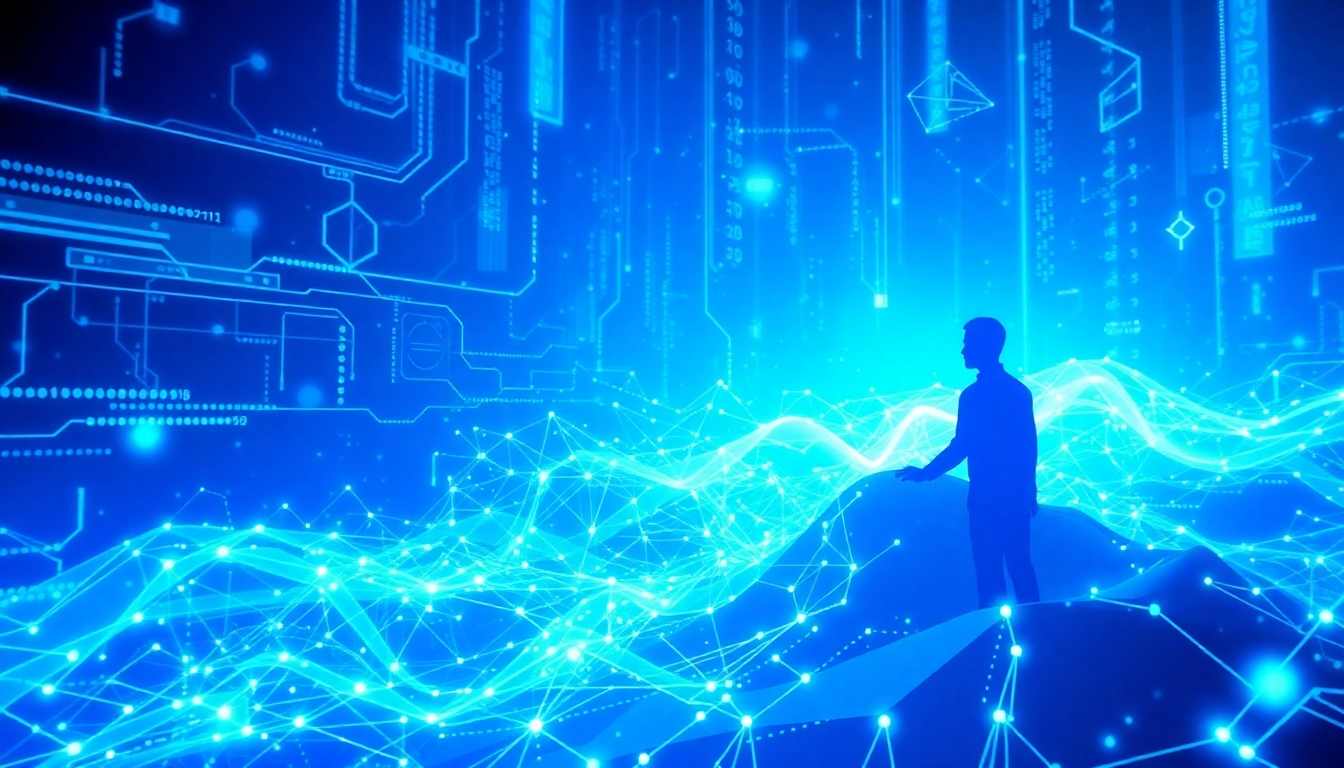
Understanding Agentic Human AI
In an era where technological advancements dictate the rhythm of progress, Agentic Human AI stands out as a formidable innovation with transformative potential for various sectors. This article explores the intricacies of Agentic Human AI—ranging from its definition and underlying technologies to its impact on the workforce and future applications. We delve into the evolutionary trajectory of this technology, examining how it operates, its benefits and challenges, and best practices for implementation.
Defining Agentic AI and Its Attributes
Agentic AI is a category of artificial intelligence that possesses the ability to act autonomously, setting and pursuing goals without requiring constant human supervision. This autonomy is key to understanding how it fundamentally differs from traditional AI models, which predominantly operate under direct human control. The essential attributes of agentic AI include:
- Autonomy: Agentic AI can make decisions and execute actions independently.
- Goal-Oriented: These systems are capable of defining objectives and motivating themselves towards achieving them.
- Adaptability: Agentic AI can adjust its behavior based on changing environments and new information, making it flexible and efficient.
- Contextual Understanding: The systems can comprehend their environment and make decisions that are suitable for the context they find themselves in.
Core Technologies Behind Agentic AI
Agentic AI leverages several foundational technologies to facilitate its autonomous operations. Among these technologies are:
- Machine Learning: Algorithms that enable the system to learn from data and improve over time without human intervention.
- Natural Language Processing (NLP): This allows the AI to understand and generate human language, facilitating interaction between machines and humans.
- Reinforcement Learning: A type of machine learning that encourages agents to take actions that maximize cumulative reward.
- Computer Vision: Empowering AI to interpret and understand visual information from the world, which is critical for applications in autonomous vehicles and surveillance systems.
Historical Development and Milestones
The development of agentic AI traces back to early AI research in the mid-20th century. However, significant milestones include:
- 1950s: Pioneering work by Alan Turing and others on machine intelligence.
- 1997: IBM’s Deep Blue became the first machine to defeat a reigning world chess champion, showcasing autonomous decision-making capabilities.
- 2010s: The rise of deep learning, which dramatically enhanced AI’s ability to learn and adapt to complex tasks.
- 2020s: The emergence of frameworks that facilitate more sophisticated real-world applications of agentic AI, including context-aware systems that autonomously adjust their behavior.
How Agentic Human AI Operates
The Mechanisms of Autonomous Decision-Making
At the heart of agentic AI is its decision-making mechanism, which employs various algorithms and models. These mechanisms include:
- Data Collection: Continuous data gathering from multiple sources to keep systems updated on the current environment.
- Data Processing: Use of advanced algorithms to analyze data, discern patterns, and extract actionable insights.
- Action Selection: Based on learned preferences and situational analysis, the AI determines the best course of action among various alternatives.
Real-World Applications and Case Studies
The impact of agentic AI spans numerous industries. Below are examples of its applications:
- Healthcare: Intelligent systems that autonomously analyze medical images and provide diagnostic suggestions, as seen in platforms like Zebra Medical Vision.
- Finance: Automated trading systems that evaluate market conditions and execute trades with minimal human oversight, such as those developed by firms like QuantConnect.
- Manufacturing: Production line robots using agentic AI to adjust operations in real-time based on production data, improving efficiency and reducing downtime.
- Customer Service: Chatbots equipped with NLP capabilities manage customer queries autonomously and provide solutions, seen in platforms like Drift.
AI Adaptability in Complex Environments
The adaptability feature of agentic AI enables these systems to operate effectively in dynamic and uncertain environments. Adaptability is realized through:
- Real-Time Learning: Continuous updates based on immediate feedback from the environment.
- Scenario Simulation: Creating and testing various hypotheses to forecast the consequences of specific actions.
- Multi-Agent Systems: When collaborating with other intelligent agents, agentic AI can adjust strategies based on interactions and collective goals.
Benefits and Challenges of Implementing Agentic Human AI
Advantages for Businesses and Productivity
Incorporating agentic AI within organizations presents numerous advantages:
- Increased Efficiency: By automating routine tasks, businesses free up human resources to focus on more complex responsibilities.
- Cost Reduction: Minimized operational costs through efficient process automation and reduced error rates.
- Enhanced Decision-Making: AI provides data-driven insights that help organizations make informed decisions swiftly.
- Scalability: Agentic AI systems can scale operations effectively to address fluctuating business needs.
Potential Ethical and Operational Challenges
Despite the benefits, implementing agentic AI is accompanied by challenges that organizations must address, such as:
- Ethical Considerations: The potential for bias in decision-making and concerns about accountability and transparency in autonomous actions.
- Security Risks: Increased vulnerabilities to attacks as reliance on AI systems grows, requiring robust cybersecurity measures.
- Job Displacement: The fear that automation will eliminate jobs, necessitating strategies for workforce transition and upskilling.
Strategies to Overcome Implementation Barriers
To successfully integrate agentic AI, organizations can adopt several strategies:
- Stakeholder Engagement: Involving all stakeholders in the design and implementation phases to address concerns from the outset.
- Adopting Ethical AI Frameworks: Developing clear ethical guidelines and standards for the use of AI technologies within the organization.
- Continuous Monitoring: Implementing systems for ongoing evaluation of the AI’s performance and its impacts on personnel and operations.
The Future of Work with Agentic Human AI
Shifts in Job Roles and Responsibilities
The rise of agentic AI is poised to redefine job roles within organizations. Many positions will transform rather than disappear entirely, leading to:
- New Roles: Positions focused on AI management, oversight, and ethical considerations will emerge alongside traditional roles.
- Shift Towards Strategic Focus: Employees will focus more on strategic decision-making rather than operational tasks, allowing for a more fulfilling work experience.
- Collaborative Workspaces: Environments fostering collaboration between humans and AI will become commonplace, encouraging mixed teams of AI and humans to tackle challenges.
Collaboration Between Humans and AI
Successful integration of agentic AI hinges on effective collaboration between humans and machines. This synergy can lead to:
- Enhanced Creativity: AI can handle data analysis, leaving humans to generate creative ideas and strategies.
- Improved Problem Solving: Together, humans and AI can tackle complex problems by combining cognitive capabilities with data processing power.
Predictions for AI-Enhanced Workspaces
The future workspace will likely feature:
- Smart Offices: Equipped with AI systems that can optimize energy use, predict maintenance needs, and adjust environments for comfort and productivity.
- Flexible Work Arrangements: AI-driven tools will facilitate remote work and collaboration across different locations seamlessly.
Best Practices for Harnessing Agentic Human AI
Implementing AI Ethically and Responsibly
Organizations must prioritize ethical considerations to guide the deployment of agentic AI. Best practices include:
- Building Transparency: Providing clear communication about AI functionalities and decision-making processes.
- Preempting Bias: Developing protocols to identify and mitigate bias in AI algorithms.
Measuring Performance and Outcomes
To gauge the effectiveness of agentic AI systems, businesses should adopt metrics such as:
- Efficiency Gains: Measuring time and cost reductions resulting from AI-driven automation.
- User Satisfaction: Assessing stakeholder satisfaction and user experience with AI applications.
Continuous Learning and AI Adaptation Strategies
For businesses to remain competitive, continuous learning and adaptation of AI systems are essential. Strategies include:
- Regular Training: Ensuring AI systems are updated with new data and scenarios to enhance accuracy.
- User Feedback Mechanisms: Implementing channels for providing feedback on AI outputs to facilitate iterative improvements.
Leave a Comment