Unlocking Insights: How AI Sentiment Analysis Transforms Business Decisions
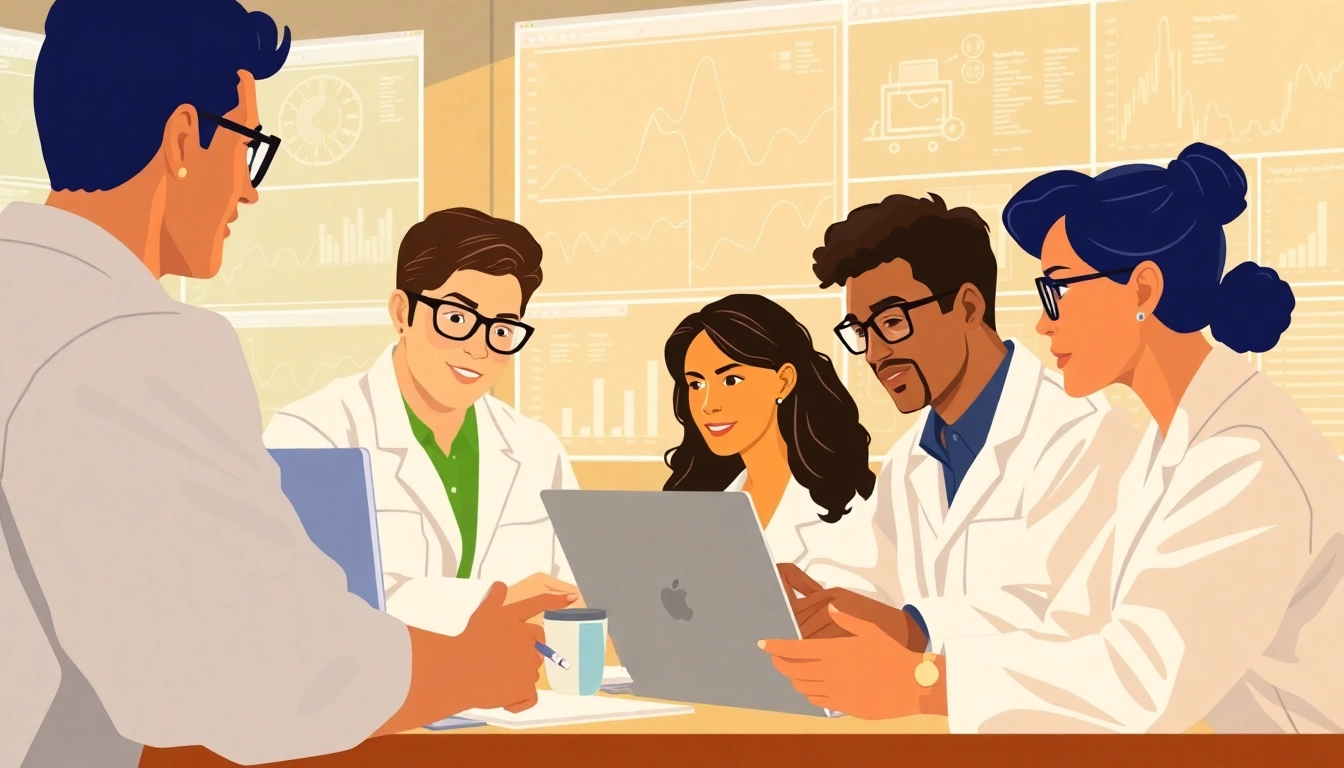
Understanding AI Sentiment Analysis
What is AI Sentiment Analysis?
AI sentiment analysis is a computational method of examining vast amounts of text data to determine and categorize the emotional tone behind it. This process utilizes advanced algorithms to classify sentiments as positive, negative, neutral, or even on a more complex emotional scale comprising various feelings like joy, anger, or surprise. In the context of a digital world filled with reviews, social media posts, emails, and customer interactions, accurately interpreting these sentiments can provide insights into consumer behavior, brand perception, and market trends. For more in-depth insight into AI sentiment analysis, you can explore AI sentiment analysis techniques and applications.
The Importance of AI Sentiment Analysis in Today’s Market
The importance of AI sentiment analysis cannot be overstated in the modern marketplace. Businesses across various sectors utilize sentiment analysis to inform marketing strategies, enhance customer support, monitor brand reputation, and improve product development. Understanding how customers feel about a product or service allows businesses to adapt quickly, ensuring they meet the evolving demands of their clientele.
For instance, real-time sentiment analysis can alert companies to a surge in negative sentiment regarding a product, enabling swift corrective actions. Furthermore, it can enhance personalization in marketing efforts, as brands can tailor their messaging based on the sentiments and preferences of their customers.
Key Techniques in AI Sentiment Analysis
Various techniques underpin AI sentiment analysis, which include:
- Natural Language Processing (NLP): This is the backbone of sentiment analysis, allowing machines to understand, interpret, and generate human language. NLP techniques help in tokenization, part-of-speech tagging, and parsing.
- Machine Learning (ML) Algorithms: Typically employed to train models to predict sentiments based on labeled data. Common algorithms include Support Vector Machines, Naive Bayes, and Neural Networks.
- Lexicon-based Approaches: These involve using a predefined list of words associated with positive or negative sentiments to score the text.
How AI Sentiment Analysis Works
Natural Language Processing and Its Role
NLP plays a critical role in sentiment analysis, enabling machines to process and understand text data. Through various stages, such as tokenization, where text is divided into words or phrases, and stemming, which reduces words to their base form, NLP prepares textual data for analysis. This preprocessing phase is essential since raw text can include numerous ambiguities.
For instance, the word “happy” might appear in a sentence with other words that could create a different sentiment. Hence, context is vital; thus, advances in NLP, particularly with the advent of models like BERT (Bidirectional Encoder Representations from Transformers), have significantly improved sentiment analysis accuracy.
Machine Learning Algorithms for Sentiment Detection
Machine learning algorithms enhance sentiment analysis by identifying patterns in data. Some of the popular ML algorithms used for sentiment detection are:
- Logistic Regression: A statistical method for binary classification models that estimate probabilities of a sentiment being positive or negative.
- Random Forest: A robust method that creates a multitude of decision trees and aggregates their predictions.
- Deep Learning Models: Such as LSTMs (Long Short-Term Memory networks) or CNNs (Convolutional Neural Networks), these models are particularly effective in capturing contextual information in text.
Integrating AI Sentiment Analysis with Data Sources
For effective sentiment analysis, integration with various data sources is crucial. These can include social media platforms, customer feedback forms, online reviews, and even call center transcriptions. Each data source provides a different perspective on customer sentiment.
Tools and platforms like Twitter API, Google Trends, and sentiment analysis software such as IBM Watson or Microsoft Azure can facilitate gathering and analyzing this data efficiently.
Applications of AI Sentiment Analysis
Enhancing Customer Engagement and Support
One of the primary applications of AI sentiment analysis lies in enhancing customer engagement. Companies leverage sentiment insights to foster meaningful interactions during customer support. For example, AI chatbots equipped with sentiment analysis capabilities can assess customer emotions in real-time and respond accordingly, improving user experience and satisfaction.
Understanding customer sentiment also allows businesses to personalize communication, targeting users with tailored messages and offers based on their expressed feelings or opinions.
Utilizing Sentiment Analysis for Brand Monitoring
Brand monitoring is another vital application of sentiment analysis. Organizations can use sentiment metrics to track public perception in real-time. By employing sentiment analysis tools, companies can gauge how their brand is viewed following product launches, advertising campaigns, or even during crises.
For instance, a sudden spike in negative sentiment after a marketing campaign might indicate that the message was poorly received, prompting a reevaluation of strategy.
Case Studies: Businesses Employing AI Sentiment Analysis
Several leading companies have effectively implemented AI sentiment analysis to drive their business strategies. Notably, global brands like Starbucks and Nike use sentiment analysis to gauge customer reactions and enhance their marketing strategies. Starbucks, for example, analyzes social media comments and reviews to adjust its menu offerings and improve store services based on customer feedback.
Furthermore, proactive brands in crisis management utilize sentiment analysis to respond swiftly to adverse reactions from the public, ensuring they address concerns before they escalate.
Challenges in AI Sentiment Analysis
Data Quality and Accuracy Issues
While AI sentiment analysis offers valuable insights, data quality remains a significant challenge. Inaccurate or biased data can lead to misleading sentiment interpretations. Textual nuances, slang, and sarcasm often complicate analysis, leading to misclassification of sentiments.
To overcome these challenges, organizations should focus on collecting clean, representative data and employing advanced NLP techniques that understand context better.
Bias and Ethical Considerations
AI sentiment analysis also faces ethical challenges, particularly around bias. If training data reflects certain biases, the models may inadvertently perpetuate or amplify these biases in analysis outcomes. This can lead to unfair treatment of certain demographic groups based on prejudiced interpretations of sentiment.
To combat this, organizations should rigorously evaluate their training datasets for bias and ensure diversity in the data to create more equitable sentiment analysis tools.
Overcoming Technical Barriers in Implementation
Implementing AI sentiment analysis can be fraught with technical barriers, such as the need for advanced computational resources, technical expertise, and integration with existing systems. Many organizations may struggle to implement complex ML models due to the high costs associated.
To address these challenges, companies can adopt cloud-based solutions which provide access to powerful sentiment analysis tools without the need for massive infrastructure investments. Moreover, utilizing pre-trained models and APIs can simplify the implementation process.
Future of AI Sentiment Analysis
Emerging Trends in AI Sentiment Analysis Tools
The future of AI sentiment analysis is poised for significant evolution, driven by technological advancements and growing data availability. Key trends include:
- Real-Time Analysis: The growing demand for instant insights will push sentiment analysis tools to process data in real-time, enabling immediate responses to changing public sentiments.
- Multi-Language Support: As businesses expand globally, sentiment analysis tools are increasingly integrating multi-language capabilities, allowing brands to monitor sentiment across diverse markets.
- Sentiment Analysis Visualization: Better visualization tools will become critical, as organizations seek to convey sentiment analysis results to stakeholders in understandable formats.
Impact of Generative AI on Sentiment Analysis
Generative AI is set to transform sentiment analysis by enhancing the depth of understanding in sentiment detection. By employing generative models, businesses can not only analyze sentiment but also predict sentiments based on evolving linguistic trends and contexts, improving the relevance of analysis over time. This capability enables organizations to stay ahead of shifts in public opinion, tailoring their strategies effectively.
Predictions for AI Sentiment Analysis in Various Industries
As industries become more data-centric, the applications of AI sentiment analysis will expand. Retail, entertainment, healthcare, and finance are examples of sectors that stand to gain significantly. Retailers will leverage sentiment insights to tailor shopping experiences and inventory management, while healthcare providers might use sentiment analysis to gauge patient satisfaction and improve care services.
Additionally, the finance sector could utilize sentiment analysis to monitor market reactions to news, allowing businesses to make better-informed investment decisions.
Leave a Comment